Pubblicazioni
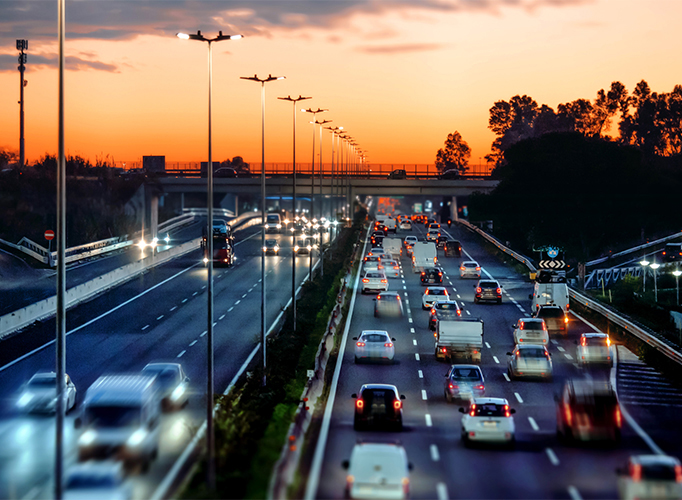
BRIEFS
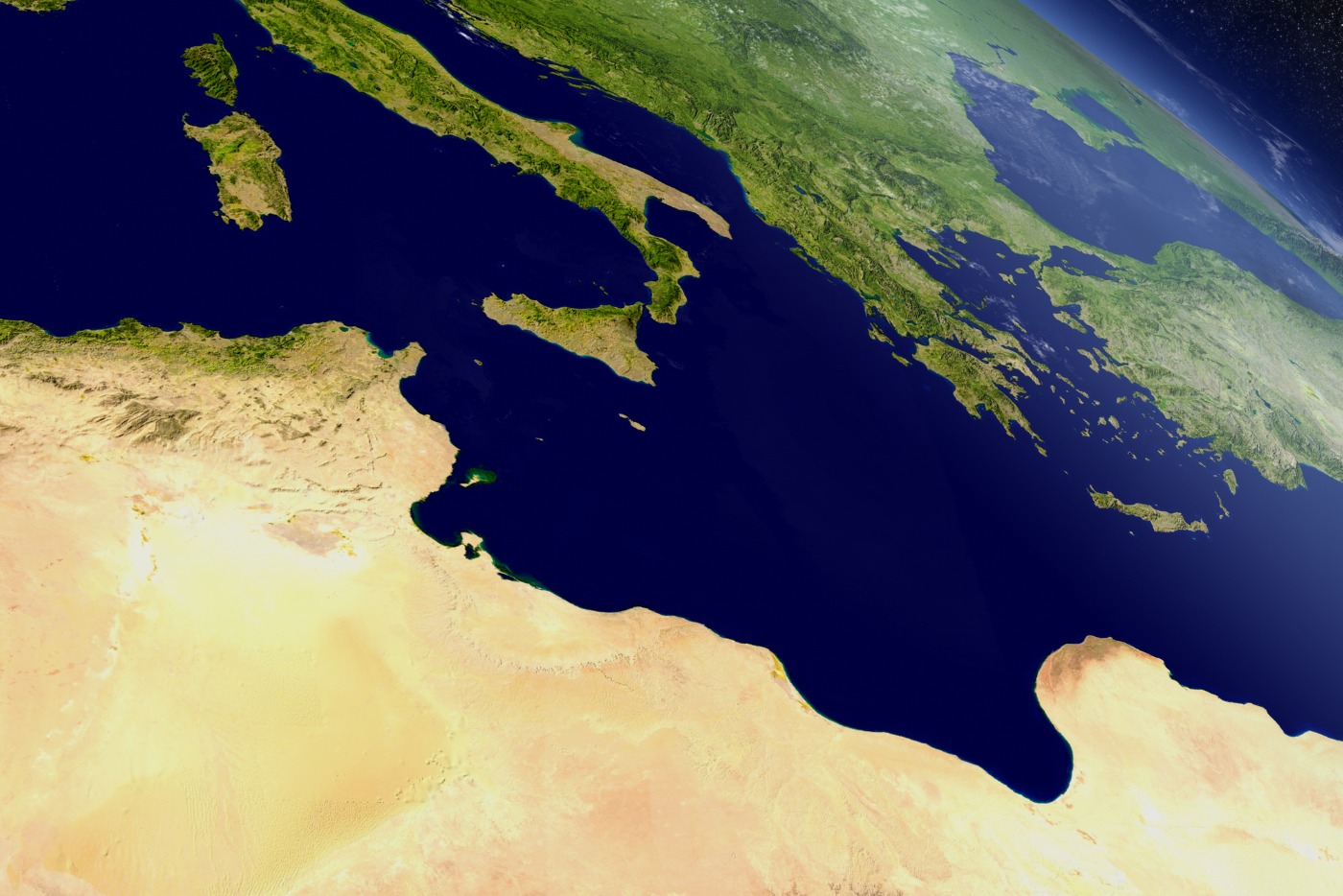
JOURNAL ARTICLES
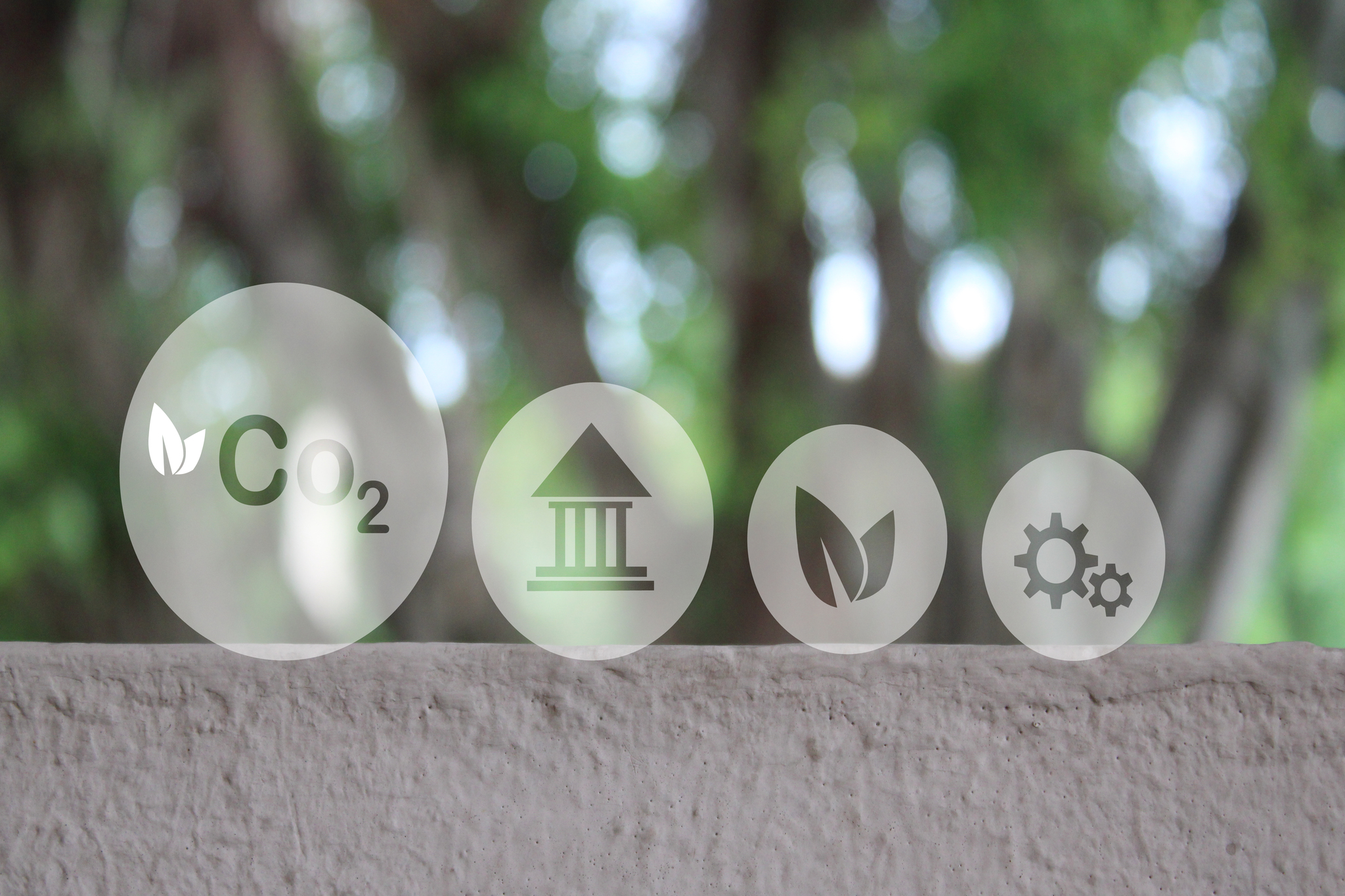
WORKING PAPERS
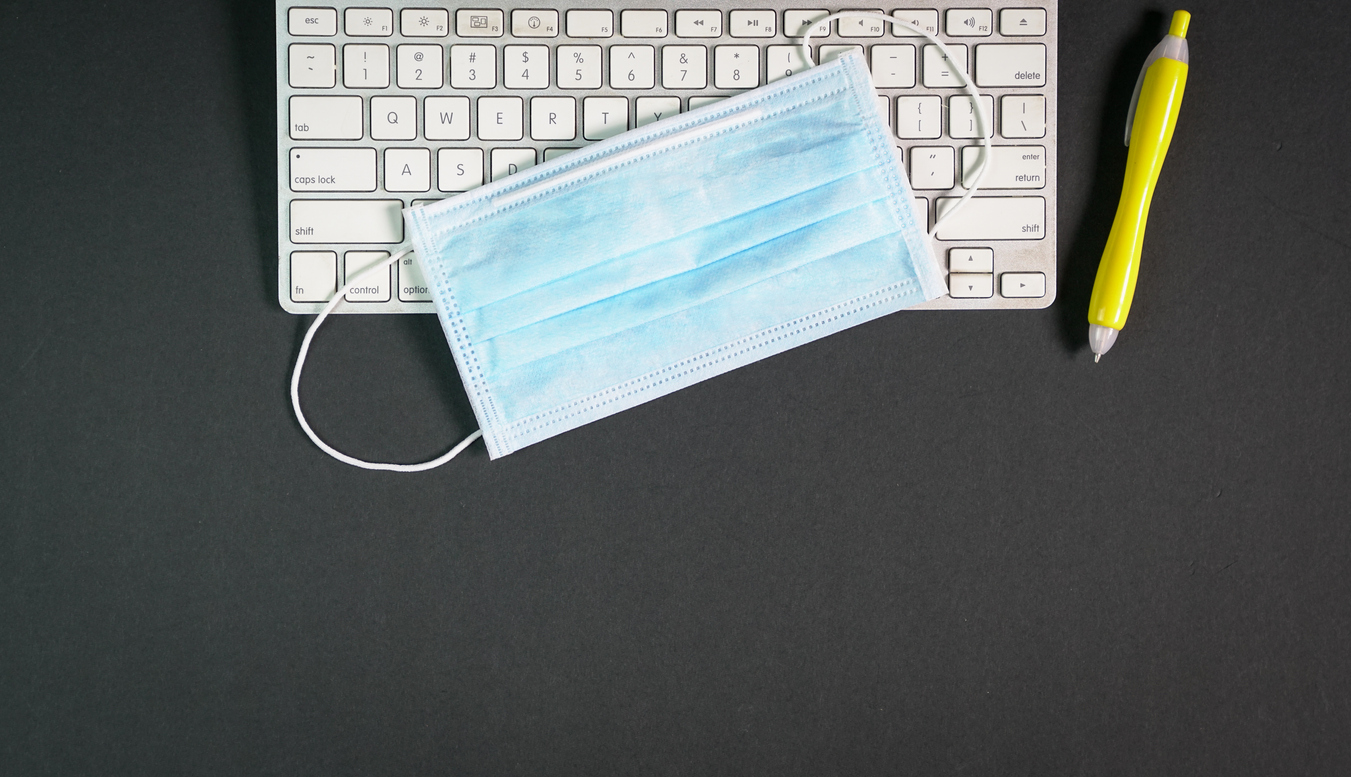
WORKING PAPERS
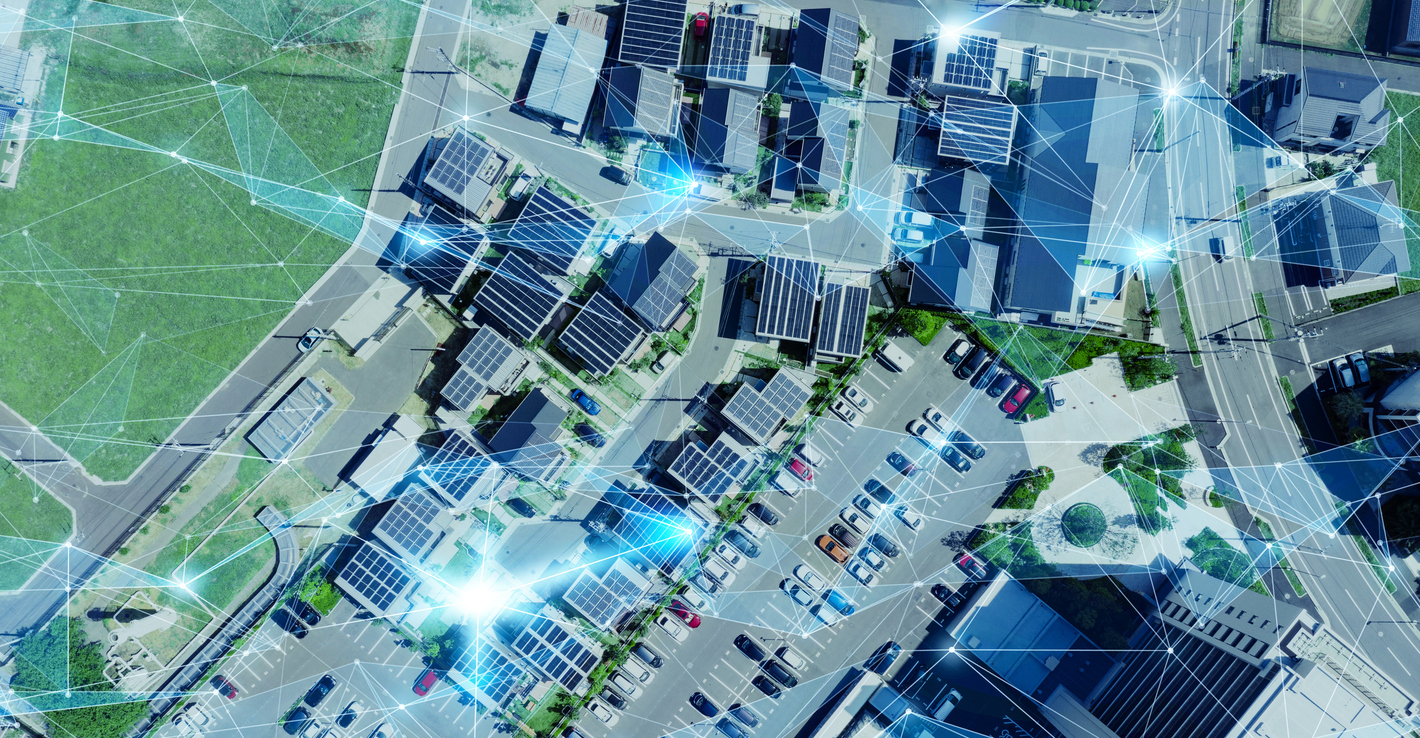
LIBRI
Eventi
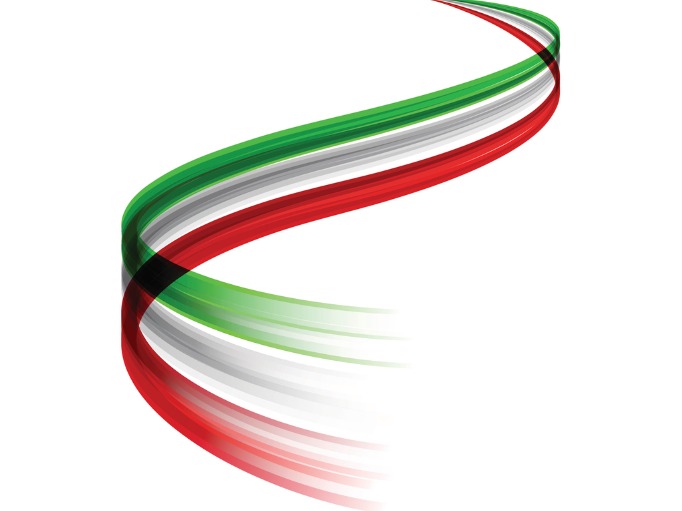
VIDEO | CONFERENZE FEEM
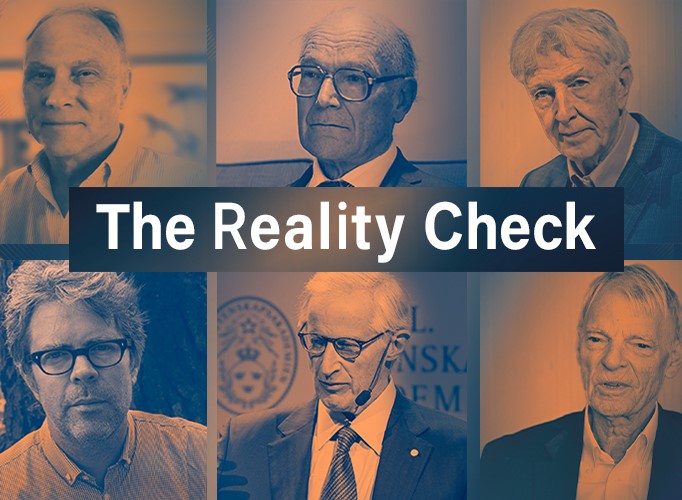
THE REALITY CHECK 2024
Resta aggiornato.
Iscriviti alla newsletter di FEEM
Entra nella community di FEEM.
Seguici su LinkedIn
Equilibri Magazine
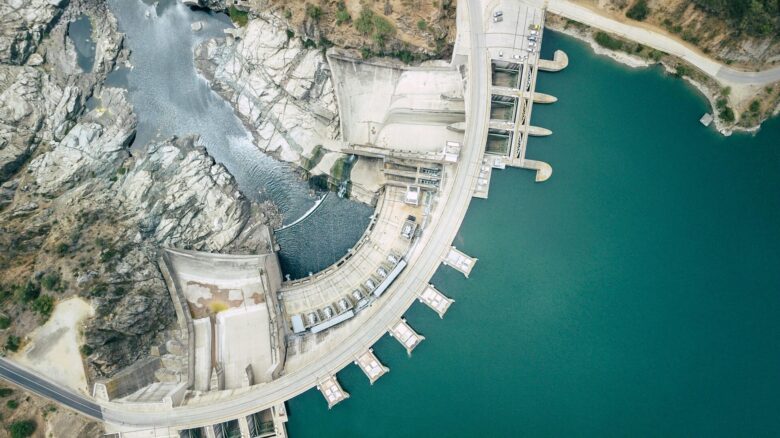